Nvidia’s latest chips for AI are the GeForce RTX 40 Series graphics cards, released in January 2024. These include the RTX 4090, 4080 Super, 4070 Ti Super, and 4070.
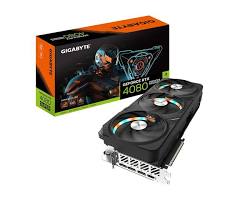
Gigabyte GeForce RTX 4080 Super Gaming OC Graphics Card
They are powered by the NVIDIA Ada Lovelace architecture, which delivers significant improvements in performance and efficiency over the previous generation. They also feature new fourth-generation Tensor Cores and DLSS 3 technology for improved AI performance.
Here is a brief comparison of the four RTX 40 Series GPUs:
Feature | RTX 4090 | RTX 4080 Super | RTX 4070 Ti Super | RTX 4070 |
---|---|---|---|---|
Video Memory | 24 GB | 16 GB | 12 GB | 12 GB |
Thermal Design Power | 450 W | 320 W | 280 W | 220 Wpen_spark |
The Nvidia RTX 4090 series shines in two major areas:
- High-Performance Gaming: The raw power of these cards makes them ideal for running the latest games at the highest resolutions (like 4K and 8K) with all the graphical bells and whistles turned on, including ray tracing and DLSS 3 for impressive image quality and performance.
- Demanding Creative Workflows: The 4090 series caters to professional content creators who work with applications like:
- 3D Rendering: These cards can significantly accelerate rendering times in applications like Blender or Maya, allowing artists to iterate faster and work on more complex projects.
- Video Editing: The improved encoding and decoding capabilities can streamline the editing workflow for high-resolution videos, especially with codecs like AV1. Applications like Adobe Premiere Pro and DaVinci Resolve benefit greatly from this.
- Scientific Computing: Fields like medical research, engineering simulations, and financial modeling can leverage the AI capabilities of these cards to perform complex calculations faster.
Here’s a breakdown of why the RTX 4090 series is a good fit for these applications:
- High Performance: The Ada Lovelace architecture and increased CUDA cores compared to previous generations translate to faster processing of demanding tasks.
- Large Video Memory: The 12GB to 24GB of GDDR6X memory allows for handling large datasets and complex textures used in creative applications.
- AI Acceleration: The 4th generation Tensor Cores are specifically designed for AI tasks, speeding up features like image and video processing, content creation, and scientific computing.
- DLSS 3: This technology uses AI to upscale images, allowing for high-resolution visuals without sacrificing performance, which is beneficial for both gamers and creators.
While the entire RTX 40 series is powerful, the RTX 4090 stands out as the most capable option, especially for users working with massive datasets or ultra-high-resolution projects.
New Chips from Nvidia soon to be released:
Nvidia announced a new AI chip platform, the Blackwell platform, at their GTC conference in March 2024. While the RTX 40 series you previously inquired about are powerful for AI tasks, the Blackwell platform is designed specifically for AI workloads and promises significant advancements.
Some key details about the upcoming Blackwell platform:
- Blackwell GPUs (B100): These are next-generation AI accelerators featuring a new architecture and innovations like 4-bit floating point capabilities for efficient AI inference.
- Grace CPU: This central processing unit integrates with the Blackwell GPUs for a powerful combined processing package.
- Grace Blackwell Superchip (GB200): This combines two B100 GPUs with a Grace CPU in a single package for maximum performance.
The entire platform is designed to excel at running large language models (LLMs) and other complex AI applications. These chips are not yet available to the public, but the debut chip, the GB200, is expected to be released later in 2024.
Chips From Other Manufacturers that Compete with Nvidida::
While Nvidia is a major player in AI chips, there are several other companies developing competitive options:
1. AMD: Their main competitor to Nvidia in the general GPU market is AMD. While their latest generation of GPUs aren’t specifically designed for AI like Nvidia’s Blackwell platform, they can still be powerful options for AI workloads. AMD is also rumored to be working on their own AI-focused chips in the future.
2. Intel: While Intel has traditionally focused on CPUs, they’re entering the AI chip game with their Intel Ponte Vecchio (PVC) chips. These are designed for high-performance computing and AI workloads, offering competition to Nvidia’s data center solutions.
3. Google: TPUs (Tensor Processing Units) are Google’s custom-designed AI accelerators. They are particularly strong for training large language models like me, and Google offers them through their Google Cloud Platform. Their latest TPU version is the Trillium, but expect them to continue innovating in this area.
4. Apple: While details are scarce, Apple is rumored to be developing their own AI chip, the M4, specifically designed for their Mac computers. This could integrate tightly with their existing M-series chips to offer strong AI performance for tasks on Apple devices.
5. Cerebras: This company focuses on building giant AI chips specifically designed for training massive neural networks. Their WSE-2 chip boasts superior processing power for certain tasks, though it comes with a much higher price tag and power consumption compared to other options.
These are just a few examples, and the AI chip market is constantly evolving. The best option for you will depend on your specific needs and budget.